082 #mysynthetic
Not to be confused with synthetic biology, when we talk about synthetic data we are talking about data that „are not obtained by direct measurement“. This is one of the many excellent topics that were debated by students participating in the University of Bern course in Open Government Data that it is my distinct pleasure to support.
Image credit: Simon Weckert - Ubuntu - the other me!
AIcrowd.com, with the slogan „Crowdsourcing A.I. to solve real world problems“, and many other projects in the A.I. space make a poignant case for the use of open ecosystems to train neural networks based on synthetic data sources, and to generate new ones. What is the interplay of open and synthetic data?
When we think about polished, published, public data, we think about many of the same things that are thought about in creating high quality data products. I have not seen much conversation about this yet in our community - and I think this is a good time to start it.
First of all, because "mock" or "fake" data is a very important topic in terms of both fighting the bad - misinformation, manipulation, misrepresentation - and as a powerful instrument to accelerate the good - bootstrapping, prototypes, A.I. at the service of social issues, there are a lot of uses for high-quality, semi-random information. And - as always - risks.
This is in opposition to data where we learn on real datasets, of real people, often collected without consent and then make "synthetic data" that often leaks private information!
— katharine jarmul (@kjam) March 23, 2022
See: https://t.co/D7idLIN9EF for more.
Perhaps a dataset to track and evaluate such sources could be a good starting point?

Please join the discussion at the online forum:
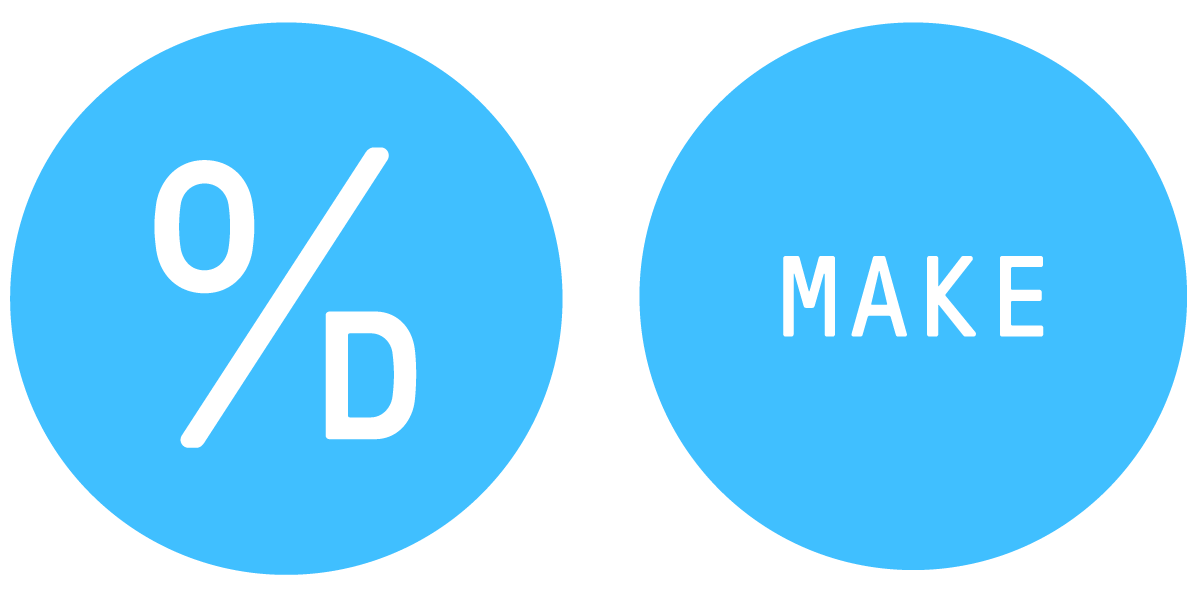
Or at Opendata.ch/2022 - where I have pitched this topic for a discussion in the public space.
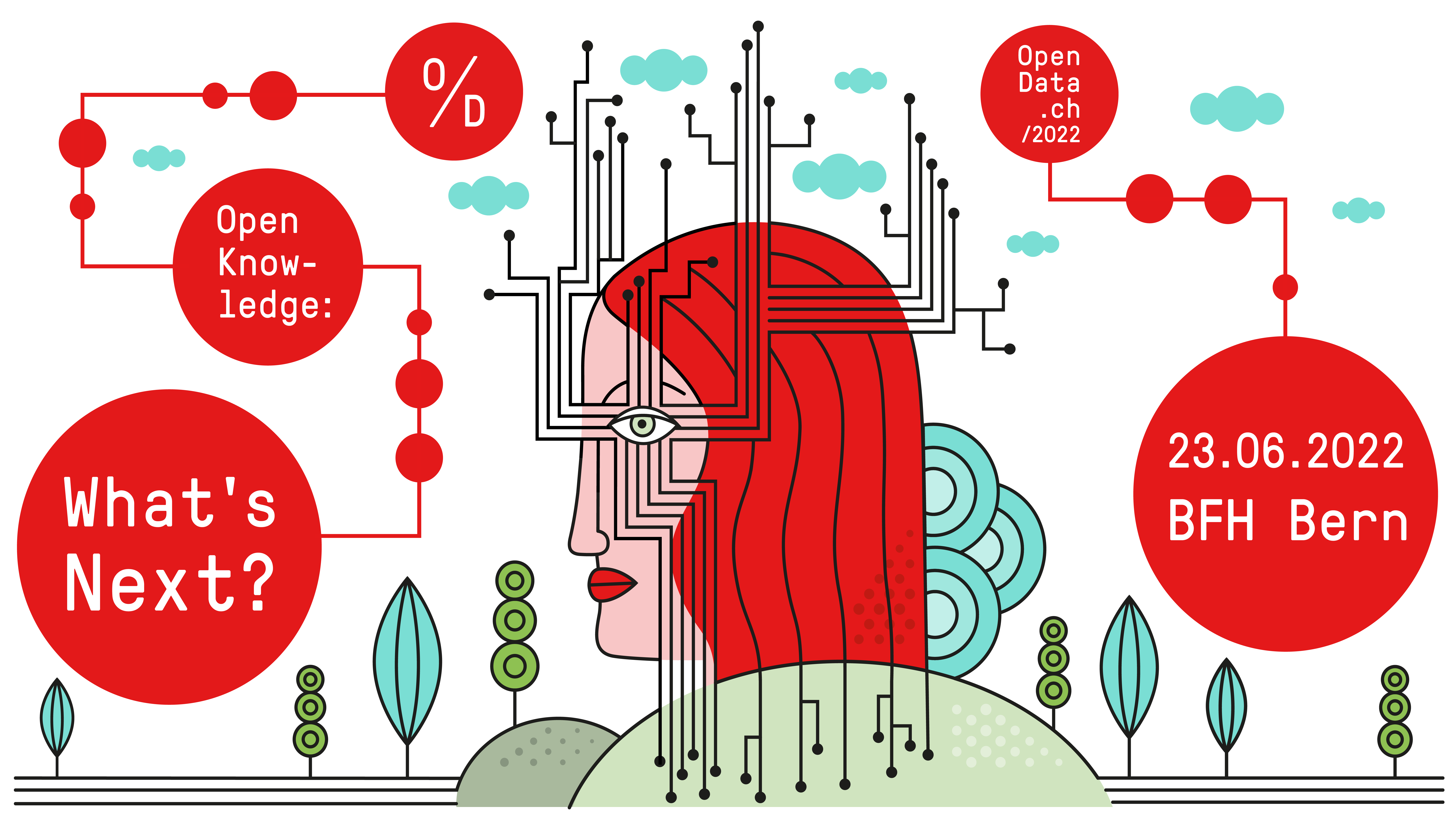